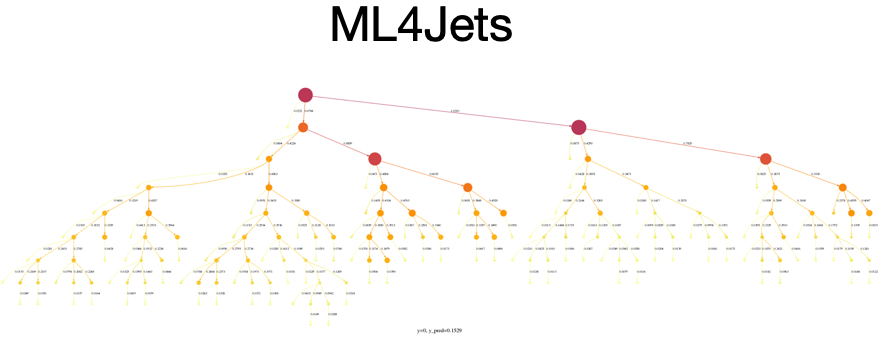
Machine Learning is having a major impact in jet physics. It is empowering powerful taggers for boosted (W, Z, H, top) jets as well as flavor tagging.
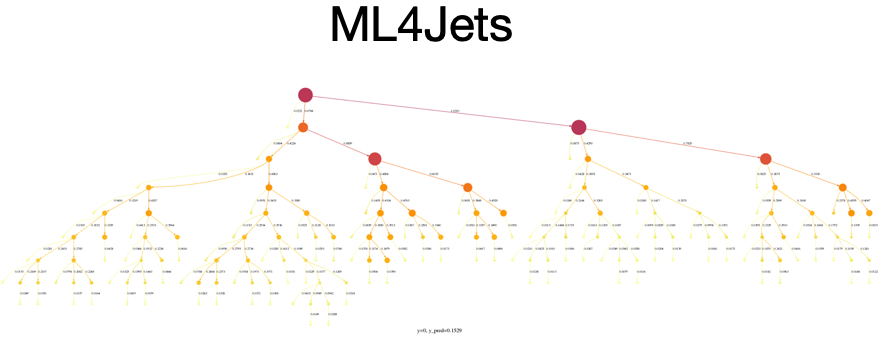
TreeNiN
The QCD-aware recursive neural networks developed as part of DIANA/HEP (Louppe et al. 2017 which leverage an analogy to natural language processing were extended to include a network-in-network. The TreeNiN method (in the table below), achieves excellent performance with orders of magnitude fewer parameters than the other top performing techniques. This pytorch implementation can be found in this repository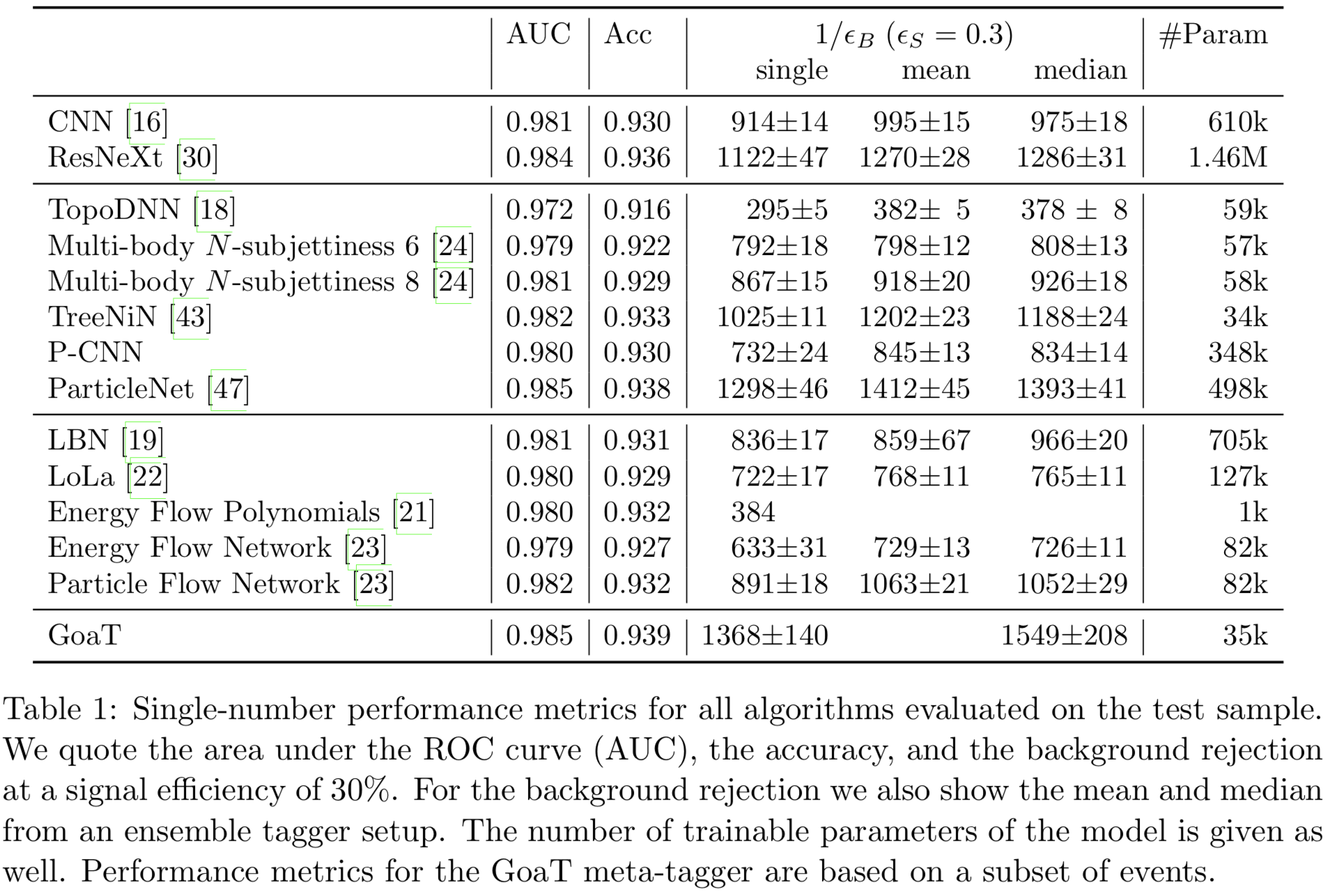
Top Tagging Comparison
At ML4Jets 2018 a top-tagging comparison was initiated, resulting in ``The Machine Learning Landscape of Top Taggers''. The table shows a comparison of the methods. Irina Espejo, Sebastian Macaluso, and Heiko Mueller are using docker containers, yadage workflows, and REANA to automate and streamline such benchmark studies.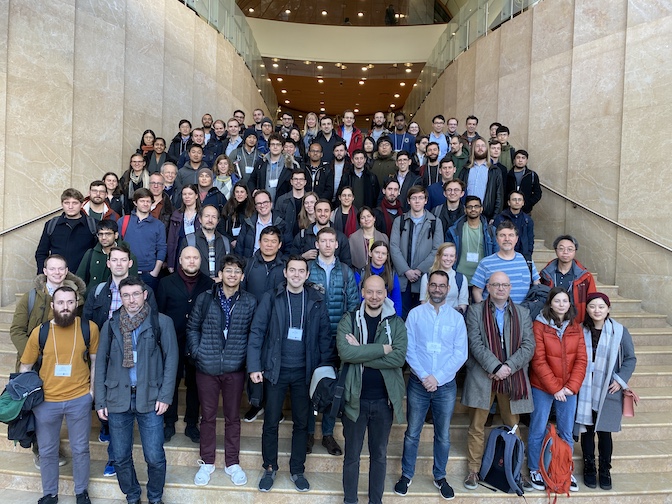
ML4Jets workshop
NYU hosted ML4Jets 2020 focusing on this topic, which was the third in the series of workshops, following ML4Jets 2018 at FermiLab and ML4Jets 2017 at Berkeley.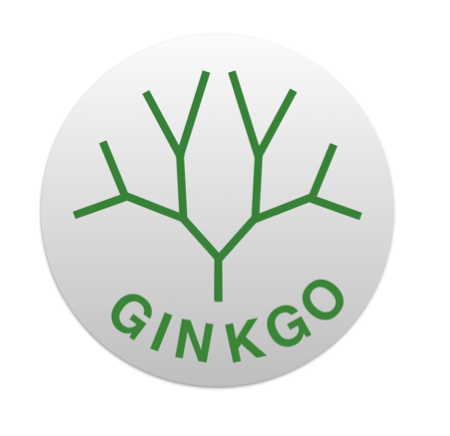
Ginkgo: growing convergent research
More recently, Sebastian Macaluso and Kyle Cranmer have worked to reframe many problems in jet physics in statistical terms and connect the parton shower to latent variable models. In order to ease collaboration with computer scientists, they have developed Ginkgo, a simplified parton shower model written in pyro.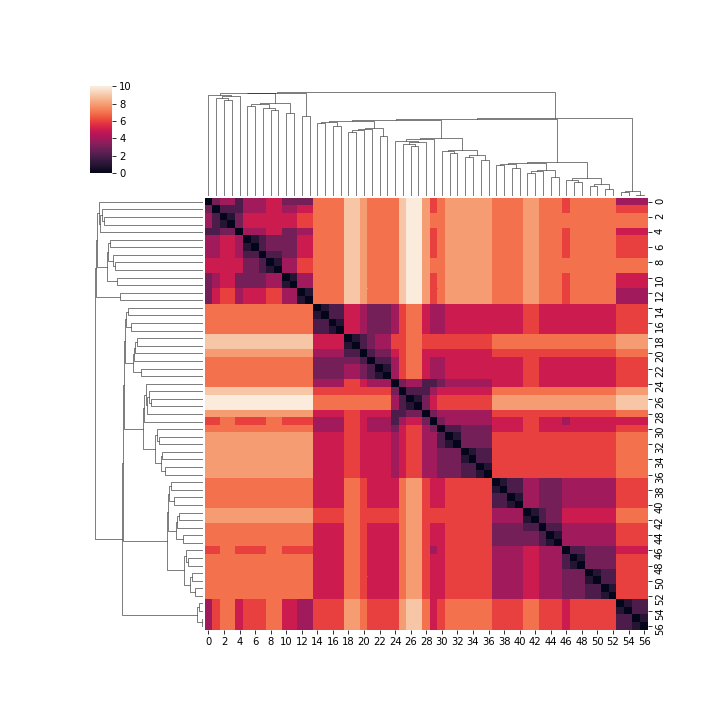
Visualizing Jet Clustering
In order to aid research into jet physics, Sebastian Macaluso, Kyle Cranmer, and Duccio Pappadopulo have developed new visualization tools to compare various jet clustering algorithms. See the VisualizeBinaryTrees repository in GitHub.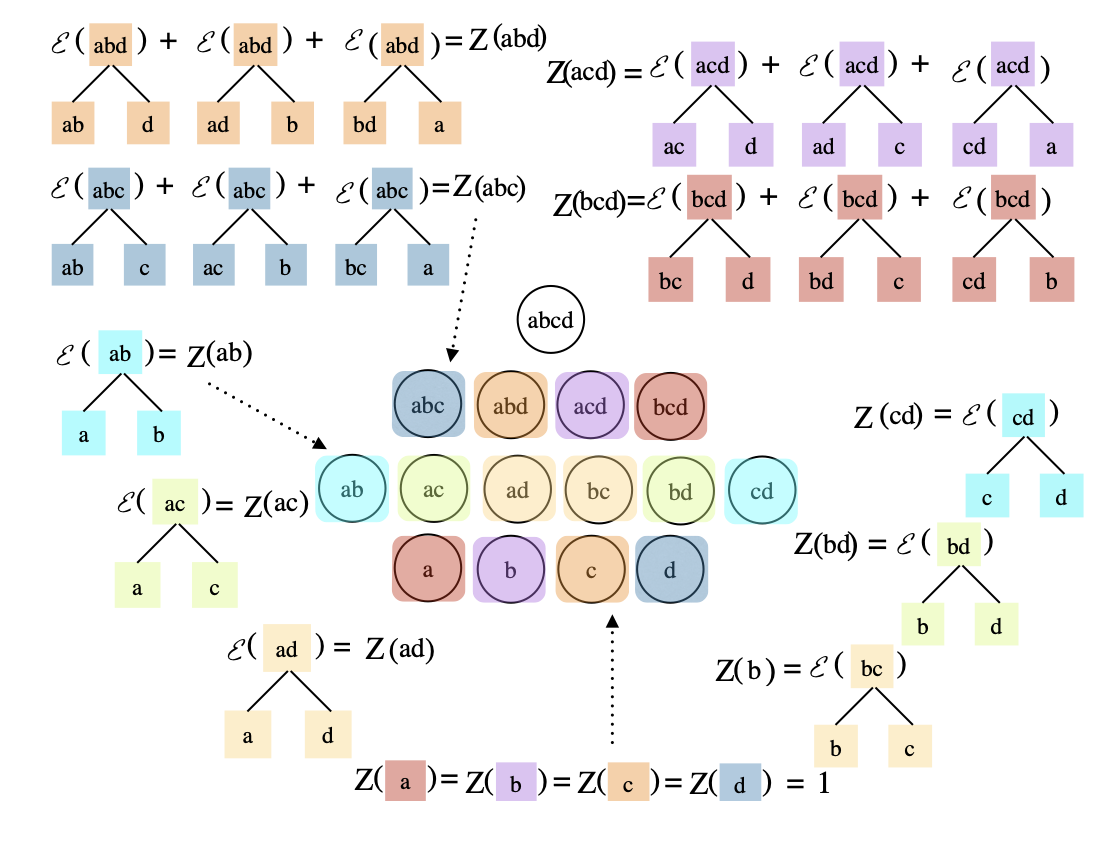
Hierarchical Clustering: use-inspired research
Hierarchical clustering is a common clustering approach for gene expression data. Within particle physics hierarchical clusterirng appears in the context of jets -- the most copiously produced objects at the Large Hadron Collider. One challenge is that the number of hierarchical clusterings grows very quickly with the number of objects being clustered. IRIS-HEP researchers Sebastian Macaluso and Kyle Cranmer connected with computer scientists at U. Mass Amherst to extend a clustering algorithm they had developed for the hierarchical case. This algorithm was applied to both particle physics and cancer genomics studies in Compact Representation of Uncertainty in Hierarchical Clustering.Team
- Kyle Cranmer
- Sebastian Macaluso
- Irina Espejo
Presentations
- 8 Jul 2021 - "Thoughts on the expressive power and inductive bias of DeepSets and Tree-Based models" , Kyle Cranmer, ML4Jets
- 6 Jul 2021 - "Emerging techniques for sampling, searching, and summing over the combinatorially large space of shower histories", Sebastian Macaluso, ML4Jets 2021
- 20 May 2021 - "Reframing Jet Physics with New Computational Methods", Sebastian Macaluso, 25th International Conference on Computing in High Energy & Nuclear Physics
- 1 Mar 2021 - "Reframing Jet Physics in Probabilistic Terms", Sebastian Macaluso, CCPP Brown Bag seminar, NYU
- 21 Sep 2020 - "New Computational Techniques and Machine Learning for Jet Physics", Sebastian Macaluso, Particle Theory Seminar, Instituto de Fisica La Plata, UNLP
- 17 Jan 2020 - "Looking into Jets with Machine Learning", Sebastian Macaluso, ML4Jets 2020
- 19 Nov 2019 - "Looking into Jets with Machine Learning", Sebastian Macaluso, Particle Theory Seminar, Harvard University
- 4 Nov 2019 - "Looking into Jets with Machine Learning", Sebastian Macaluso, Data Science Tea UMass
- 1 Nov 2019 - "Looking into Jets with Machine Learning", Sebastian Macaluso, NYU Physics x ML
- 25 Sep 2019 - "Benchmarks for ML4Jets research", Sebastian Macaluso, IRIS-HEP Topical Meeting
- 24 Sep 2019 - "Looking into Jets with Machine Learning", Sebastian Macaluso, Princeton Pheno & Vino Seminar
- 23 Jul 2019 - "The Machine Learning Landscape of Top Taggers", Sebastian Macaluso, 11th International Workshop on Boosted Object Phenomenology, Reconstruction and Searches in HEP (BOOST 2019)
- 19 Jun 2019 - "SCAILFIN: Reproducible Open Benchmarks", Sebastian Macaluso, Analysis Systems Topical Meeting
- 15 Nov 2018 - "Pulling Out All the Tops with Computer Vision and Deep Learning", Sebastian Macaluso, Machine Learning for Jet Physics
Publications
- The Quantum Trellis: A classical algorithm for sampling the parton shower with interference effects, S. Macaluso and K. Cranmer, arXiv 2112.12795 (23 Dec 2021) [3 citations].
- Reframing Jet Physics with New Computational Methods, The 25th International Conference on Computing in High-Energy and Nuclear Physics, vCHEP 2021. (21 May 2021) [9 citations] [NSF PAR].
- Exact and Approximate Hierarchical Clustering Using A*, The Conference on Uncertainty in Artificial Intelligence (UAI) 2021. (14 Apr 2021).
- Hierarchical clustering in particle physics through reinforcement learning, Machine Learning and the Physical Sciences, Workshop at the 34th Conference on Neural Information Processing Systems (NeurIPS), December 11, 2020 (16 Nov 2020) [5 citations].
- Snowmass 2021 Letter of Interest: Emerging Computational Techniques for Jet Physics, Sebastian Macaluso, Kyle Cranmer, Matthew Drnevich, Johann Brehmer (New York University); Duccio Pappadopulo (N.A.); Atılım Gunes Baydin (Oxford); Matthew Schwartz (Harvard), Snowmass 2021 Letters of Interest (31 Aug 2020).
- Secondary vertex finding in jets with neural networks, J. Shlomi, S. Ganguly, E. Gross, K. Cranmer, Y. Lipman, H. Serviansky, H. Maron and N. Segol, Eur.Phys.J.C 81 540 (2021) (06 Aug 2020) [29 citations] [NSF PAR].
- Data Structures & Algorithms for Exact Inference in Hierarchical Clustering, The 24th International Conference on Artificial Intelligence and Statistics, PMLR 130:2467-2475, 2021. (26 Feb 2020) [3 citations].
- Set2Graph: Learning Graphs From Sets, Advances in Neural Information Processing Systems 34 (NeurIPS2020) (20 Feb 2020) [13 citations].
- Hamiltonian Graph Networks with ODE Integrators, A. Sanchez-Gonzalez, V. Bapst, K. Cranmer and P. Battaglia, arXiv 1909.12790 (Submitted to Machine Learning For the Physical Sciences NeurIPS2019 Workshop) (27 Sep 2019) [12 citations].
- The Machine Learning landscape of top taggers, G. Kasieczka et. al., SciPost Phys. 7 014 (2019) (26 Feb 2019) [234 citations] [NSF PAR].
- QCD-Aware Recursive Neural Networks for Jet Physics, G. Louppe, K. Cho, C. Becot and K. Cranmer, JHEP 01 057 (2019) (02 Feb 2017) [195 citations].
Recent recordings
Sukanya Krishna on Particle Graph Autoencoders for Real-Time Jet Anomaly Detection
20 Sep 2021
Jason Wong on Developing Lorentz Equivariant Graph Neural Networks for Jet Tagging
20 Sep 2021